5.17. DataFrame Mapping
Series.map()
- Map values of Series according to an input mapping or functionSeries.apply()
- Invoke function on values of SeriesDataFrame.apply()
- Apply a function along an axis of the DataFrameDataFrame.applymap()
- Apply a function to a Dataframe elementwiseDataFrame.pipe()
- Apply chainable functions that expect Series or DataFramesDataFrame.where(cond, sub)
- Replace values where the condition is FalseDataFrame.mask(cond, sub)
- Replace values where the condition is TrueSeries.str.split(regex, expand=True)
- Split strings around given separator/delimiterSeries.str.extract(regex, expand=True)
- Extract capture groups in the regex pat as columns in a DataFrameSeries.str.extractall()
- Extract capture groups in the regex pat as columns in DataFrameSeries.str.findall(regex)
- Find all occurrences of pattern or regular expression in the Series/IndexSeries.str.fullmatch(regex)
- Determine if each string entirely matches a regular expressionSeries.dt.strftime(...)
- formatted strings specified by date_format, which supports the same string format as the python standard librarySeries.dt.date
- Returns numpy array of python datetime.date objectsSeries.dt.time
- Returns numpy array of datetime.time objectsSeries.dt.timez
- Returns numpy array of datetime.time objects with timezone information
5.17.1. SetUp
>>> import pandas as pd
>>> import numpy as np
>>> np.random.seed(0)
>>>
>>>
>>> df = pd.DataFrame(
... columns = ['Morning', 'Noon', 'Evening', 'Midnight'],
... index = pd.date_range('1999-12-30', periods=7),
... data = np.random.randn(7, 4))
>>>
>>> df
Morning Noon Evening Midnight
1999-12-30 1.764052 0.400157 0.978738 2.240893
1999-12-31 1.867558 -0.977278 0.950088 -0.151357
2000-01-01 -0.103219 0.410599 0.144044 1.454274
2000-01-02 0.761038 0.121675 0.443863 0.333674
2000-01-03 1.494079 -0.205158 0.313068 -0.854096
2000-01-04 -2.552990 0.653619 0.864436 -0.742165
2000-01-05 2.269755 -1.454366 0.045759 -0.187184
5.17.2. Map
Works only on Series
Argument:
dict
,Series
, orCallable
Works element-wise on a Series
Operate on one element at time
When passed a dictionary/Series will map elements based on the keys in that dictionary/Series, missing values will be recorded as
NaN
in the outputIs optimised for elementwise mappings and transformation
Operations that involve dictionaries or Series will enable pandas to use faster code paths for better performance [5]
>>> df['Morning'].map(lambda value: round(value, 2))
1999-12-30 1.76
1999-12-31 1.87
2000-01-01 -0.10
2000-01-02 0.76
2000-01-03 1.49
2000-01-04 -2.55
2000-01-05 2.27
Freq: D, Name: Morning, dtype: float64
>>> df['Morning'].map(int)
1999-12-30 1
1999-12-31 1
2000-01-01 0
2000-01-02 0
2000-01-03 1
2000-01-04 -2
2000-01-05 2
Freq: D, Name: Morning, dtype: int64
5.17.3. Apply
Works on both Series and DataFrame
Argument:
Callable
On Series: operate on one element at time
On DataFrame: elementwise but also row / column basis
Suited to more complex operations and aggregation
The behaviour and return value depends on the function
Returns a scalar for aggregating operations, Series otherwise. Similarly for
DataFrame.apply
Has fastpaths when called with certain NumPy functions such as
mean
,sum
, etc. [5]
>>> df['Morning'].apply(int)
1999-12-30 1
1999-12-31 1
2000-01-01 0
2000-01-02 0
2000-01-03 1
2000-01-04 -2
2000-01-05 2
Freq: D, Name: Morning, dtype: int64
>>> df['Morning'].apply(lambda value: round(value, 2))
1999-12-30 1.76
1999-12-31 1.87
2000-01-01 -0.10
2000-01-02 0.76
2000-01-03 1.49
2000-01-04 -2.55
2000-01-05 2.27
Freq: D, Name: Morning, dtype: float64
5.17.4. Applymap
Works only on DataFrame
Argument:
Callable
Works element-wise on a DataFrame
Operate on one element at time
In more recent versions has been optimised for some operations
You will find
applymap
slightly faster thanapply
in some cases.Test both and use whatever works better [5]
5.17.5. Summary
Series.map
[1]:
Works element-wise on a Series
Operate on one element at time
Series.apply
[2]:
Operate on one element at time
DataFrame.apply
[3]:
Works on a row / column basis of a DataFrame
Operates on entire rows or columns at a time
DataFrame.applymap
[4]:
Works element-wise on a DataFrame
Operate on one element at time
5.17.6. Differentiation
Definition:
map
defined onSeries
only
applymap
defined onSeries
andDataFrame
apply
defined onDataFrame
only
Argument type:
map
takesdict
,Series
,Callable
apply
takesCallable
only
applymap
takesCallable
only
Behavior:
map
elementwise
apply
elementwise but is suited to more complex operations and aggregation; the behaviour and return value depends on the function
applymap
elementwise
Use Case:
map
is meant for mapping values from one domain to another, so is optimised for performance (e.g.,df['A'].map({1:'a', 2:'b', 3:'c'})
)
apply
is for applying any function that cannot be vectorised (e.g.,df['sentences'].apply(nltk.sent_tokenize)
)
applymap
is good for elementwise transformations across multiple rows/columns (e.g.,df[['A', 'B', 'C']].applymap(str.strip)
)
Footnotes [5]:
map
is optimised for elementwise mappings and transformation. Operations that involve dictionaries or Series will enable pandas to use faster code paths for better performance. When passed a dictionary/Series will map elements based on the keys in that dictionary/Series; missing values will be recorded asNaN
in the output
apply
returns a scalar for aggregating operations, Series otherwise. Note thatapply
also has fastpaths when called with certain NumPy functions such asmean
,sum
, etc.
applymap
in more recent versions has been optimised for some operations. You will findapplymap
slightly faster thanapply
in some cases. Test both and use whatever works better.
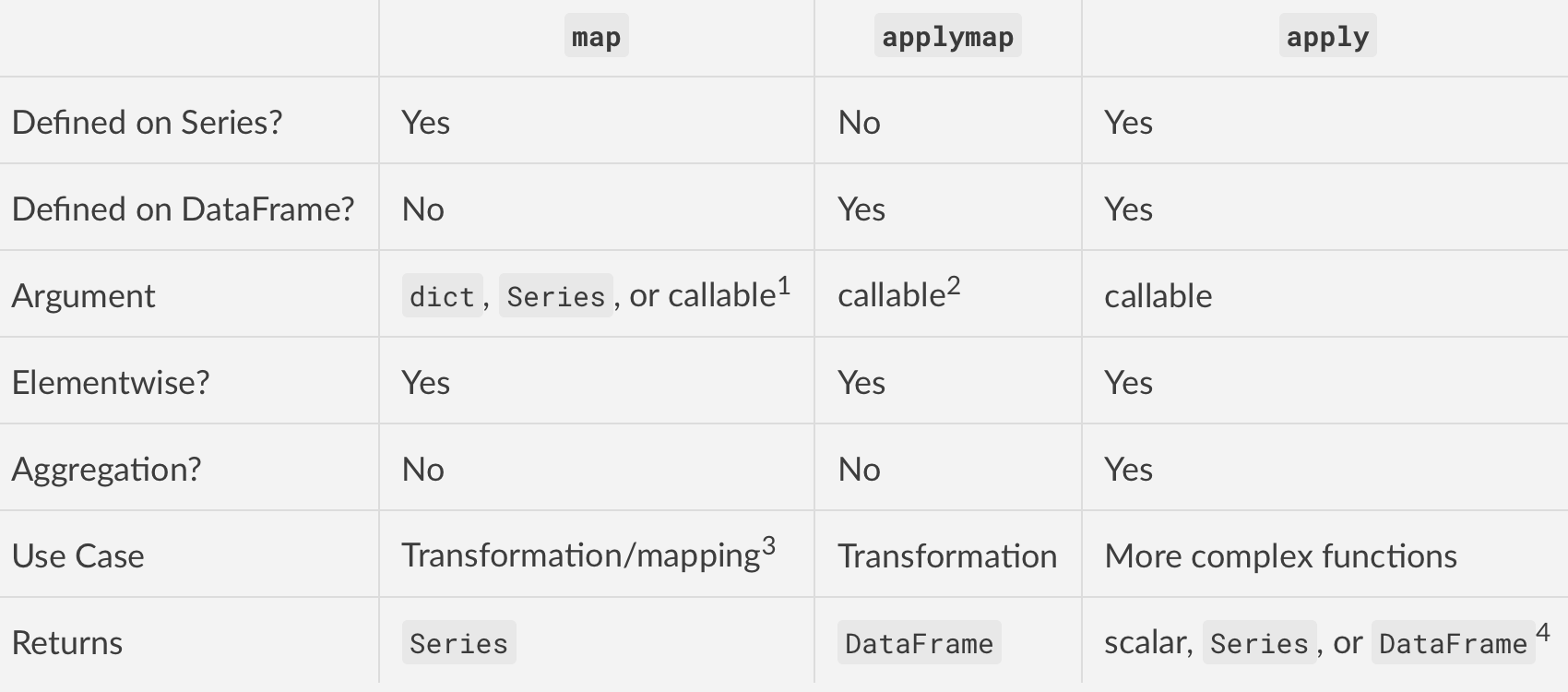
5.17.7. References
5.17.8. Assignments
# %% License
# - Copyright 2025, Matt Harasymczuk <matt@python3.info>
# - This code can be used only for learning by humans
# - This code cannot be used for teaching others
# - This code cannot be used for teaching LLMs and AI algorithms
# - This code cannot be used in commercial or proprietary products
# - This code cannot be distributed in any form
# - This code cannot be changed in any form outside of training course
# - This code cannot have its license changed
# - If you use this code in your product, you must open-source it under GPLv2
# - Exception can be granted only by the author
# %% Run
# - PyCharm: right-click in the editor and `Run Doctest in ...`
# - PyCharm: keyboard shortcut `Control + Shift + F10`
# - Terminal: `python -m doctest -v myfile.py`
# %% About
# - Name: DataFrame Mapping Split
# - Difficulty: easy
# - Lines: 5
# - Minutes: 5
# %% English
# 1. Read data from `DATA` as `df: pd.DataFrame`
# 2. Parse data in `datetime` column as `datetime` object
# 3. Split column `datetime` with into two separate: date and time columns
# 4. Run doctests - all must succeed
# %% Polish
# 1. Wczytaj dane z `DATA` jako `df: pd.DataFrame`
# 2. Sparsuj dane w kolumnie `datetime` jako obiekty `datetime`
# 3. Podziel kolumnę z `datetime` na dwie osobne: datę i czas
# 4. Uruchom doctesty - wszystkie muszą się powieść
# %% Hints
# - `pd.Series.dt.date`
# - `pd.Series.dt.time`
# %% Tests
"""
>>> import sys; sys.tracebacklimit = 0
>>> assert sys.version_info >= (3, 9), \
'Python 3.9+ required'
>>> pd.set_option('display.width', 500)
>>> pd.set_option('display.max_columns', 10)
>>> pd.set_option('display.max_rows', 10)
>>> assert result is not Ellipsis, \
'Assign result to variable: `result`'
>>> assert type(result) is pd.DataFrame, \
'Variable `result` must be a `pd.DataFrame` type'
>>> result # doctest: +NORMALIZE_WHITESPACE
id period datetime network item type duration date time
0 0 1999-11 1999-10-15 06:58:00 T-Mobile data data 34.5 1999-10-15 06:58:00
1 1 1999-11 1999-10-15 06:58:00 Orange call mobile 13.0 1999-10-15 06:58:00
2 2 1999-11 1999-10-15 14:46:00 Play call mobile 23.0 1999-10-15 14:46:00
3 3 1999-11 1999-10-15 14:48:00 Plus call mobile 4.0 1999-10-15 14:48:00
4 4 1999-11 1999-10-15 17:27:00 T-Mobile call mobile 4.0 1999-10-15 17:27:00
.. ... ... ... ... ... ... ... ... ...
825 825 2000-03 2000-03-13 00:38:00 AT&T sms international 1.0 2000-03-13 00:38:00
826 826 2000-03 2000-03-13 00:39:00 Orange sms mobile 1.0 2000-03-13 00:39:00
827 827 2000-03 2000-03-13 06:58:00 Orange data data 34.5 2000-03-13 06:58:00
828 828 2000-03 2000-03-14 00:13:00 AT&T sms international 1.0 2000-03-14 00:13:00
829 829 2000-03 2000-03-14 00:16:00 AT&T sms international 1.0 2000-03-14 00:16:00
<BLANKLINE>
[830 rows x 9 columns]
"""
import pandas as pd
DATA = 'https://python3.info/_static/phones-pl.csv'
# type: pd.DataFrame
result = ...
# FIXME: pd.to_datetime(errors='ignore') is deprecated and will raise in a future version
# %% License
# - Copyright 2025, Matt Harasymczuk <matt@python3.info>
# - This code can be used only for learning by humans
# - This code cannot be used for teaching others
# - This code cannot be used for teaching LLMs and AI algorithms
# - This code cannot be used in commercial or proprietary products
# - This code cannot be distributed in any form
# - This code cannot be changed in any form outside of training course
# - This code cannot have its license changed
# - If you use this code in your product, you must open-source it under GPLv2
# - Exception can be granted only by the author
# %% Run
# - PyCharm: right-click in the editor and `Run Doctest in ...`
# - PyCharm: keyboard shortcut `Control + Shift + F10`
# - Terminal: `python -m doctest -v myfile.py`
# %% About
# - Name: DataFrame Mapping Translate
# - Difficulty: easy
# - Lines: 5
# - Minutes: 5
# %% English
# 1. Read data from `DATA` as `df: pd.DataFrame`
# 2. Convert Polish month names to English
# 3. Parse dates to `datetime` objects
# 4. Select columns ['firstname', 'lastname', 'birthdate']
# 5. Run doctests - all must succeed
# %% Polish
# 1. Wczytaj dane z `DATA` jako `df: pd.DataFrame`
# 2. Przekonwertuj polskie nazwy miesięcy na angielskie
# 3. Sparsuj daty do obiektów `datetime`
# 4. Wybierz kolumny ['firstname', 'lastname', 'birthdate']
# 5. Uruchom doctesty - wszystkie muszą się powieść
# %% Hints
# - `pd.Series.replace(regex=True)`
# - `pd.to_datetime()`
# %% Tests
"""
>>> import sys; sys.tracebacklimit = 0
>>> assert sys.version_info >= (3, 9), \
'Python 3.9+ required'
>>> pd.set_option('display.width', 500)
>>> pd.set_option('display.max_columns', 10)
>>> pd.set_option('display.max_rows', 10)
>>> assert result is not Ellipsis, \
'Assign result to variable: `result`'
>>> assert type(result) is pd.DataFrame, \
'Variable `result` must be a `pd.DataFrame` type'
>>> result[['firstname', 'lastname', 'birthdate']] # doctest: +NORMALIZE_WHITESPACE
firstname lastname birthdate
0 Mark Watney 1994-10-12
1 Melissa Lewis 1995-07-07
2 Rick Martinez 1996-01-21
3 Alex Vogel 1994-11-15
4 Beth Johanssen 2006-05-09
5 Chris Beck 1999-08-02
"""
import pandas as pd
DATA = 'https://python3.info/_static/martian-pl.csv'
MONTHS_PLEN = {'styczeń': 'January',
'luty': 'February',
'marzec': 'March',
'kwiecień': 'April',
'maj': 'May',
'czerwiec': 'June',
'lipiec': 'July',
'sierpień': 'August',
'wrzesień': 'September',
'październik': 'October',
'listopad': 'November',
'grudzień': 'December'}
# type: pd.DataFrame
result = ...
# %% License
# - Copyright 2025, Matt Harasymczuk <matt@python3.info>
# - This code can be used only for learning by humans
# - This code cannot be used for teaching others
# - This code cannot be used for teaching LLMs and AI algorithms
# - This code cannot be used in commercial or proprietary products
# - This code cannot be distributed in any form
# - This code cannot be changed in any form outside of training course
# - This code cannot have its license changed
# - If you use this code in your product, you must open-source it under GPLv2
# - Exception can be granted only by the author
# %% Run
# - PyCharm: right-click in the editor and `Run Doctest in ...`
# - PyCharm: keyboard shortcut `Control + Shift + F10`
# - Terminal: `python -m doctest -v myfile.py`
# %% About
# - Name: DataFrame Mapping Month
# - Difficulty: easy
# - Lines: 10
# - Minutes: 8
# %% English
# 1. Read data from `DATA` as `df: pd.DataFrame`
# 2. Add column `year` and `month` by parsing `period` column
# 3. Month name must be a string month name, not a number (i.e.: 'January', 'May')
# 4. Example: if `period` column is "2015-01", then `year`: 2015, `month`: January
# 5. Run doctests - all must succeed
# %% Polish
# 1. Wczytaj dane z `DATA` jako `df: pd.DataFrame`
# 2. Dodaj kolumnę `year` i `month` poprzez sparsowanie kolumny `period`
# 3. Nazwa miesiąca musi być ciągiem znaków, a nie liczbą (i.e. 'January', 'May')
# 4. Example: jeżeli kolumna `period` jest "2015-01", to `year`: 2015, `month`: January
# 5. Uruchom doctesty - wszystkie muszą się powieść
# %% Hints
# - `Series.str.split(expand=True)`
# - `df[ ['A', 'B'] ] = ...`
# %% Tests
"""
>>> import sys; sys.tracebacklimit = 0
>>> assert sys.version_info >= (3, 9), \
'Python 3.9+ required'
>>> pd.set_option('display.width', 500)
>>> pd.set_option('display.max_columns', 10)
>>> pd.set_option('display.max_rows', 10)
>>> assert result is not Ellipsis, \
'Assign result to variable: `result`'
>>> assert type(result) is pd.DataFrame, \
'Variable `result` must be a `pd.DataFrame` type'
>>> result # doctest: +NORMALIZE_WHITESPACE
id period datetime network item type duration year month
0 0 1999-11 1999-10-15 06:58 T-Mobile data data 34.5 1999 November
1 1 1999-11 1999-10-15 06:58 Orange call mobile 13.0 1999 November
2 2 1999-11 1999-10-15 14:46 Play call mobile 23.0 1999 November
3 3 1999-11 1999-10-15 14:48 Plus call mobile 4.0 1999 November
4 4 1999-11 1999-10-15 17:27 T-Mobile call mobile 4.0 1999 November
.. ... ... ... ... ... ... ... ... ...
825 825 2000-03 2000-03-13 00:38 AT&T sms international 1.0 2000 March
826 826 2000-03 2000-03-13 00:39 Orange sms mobile 1.0 2000 March
827 827 2000-03 2000-03-13 06:58 Orange data data 34.5 2000 March
828 828 2000-03 2000-03-14 00:13 AT&T sms international 1.0 2000 March
829 829 2000-03 2000-03-14 00:16 AT&T sms international 1.0 2000 March
<BLANKLINE>
[830 rows x 9 columns]
"""
import pandas as pd
DATA = 'https://python3.info/_static/phones-pl.csv'
MONTHS_EN = ['January', 'February', 'March', 'April',
'May', 'June', 'July', 'August', 'September',
'October', 'November', 'December']
MONTHS = dict(enumerate(MONTHS_EN, start=1))
# type: pd.DataFrame
result = ...
# %% License
# - Copyright 2025, Matt Harasymczuk <matt@python3.info>
# - This code can be used only for learning by humans
# - This code cannot be used for teaching others
# - This code cannot be used for teaching LLMs and AI algorithms
# - This code cannot be used in commercial or proprietary products
# - This code cannot be distributed in any form
# - This code cannot be changed in any form outside of training course
# - This code cannot have its license changed
# - If you use this code in your product, you must open-source it under GPLv2
# - Exception can be granted only by the author
# %% Run
# - PyCharm: right-click in the editor and `Run Doctest in ...`
# - PyCharm: keyboard shortcut `Control + Shift + F10`
# - Terminal: `python -m doctest -v myfile.py`
# %% About
# - Name: DataFrame Mapping Substitute
# - Difficulty: medium
# - Lines: 10
# - Minutes: 8
# %% English
# 1. Read data from `DATA` as `df: pd.DataFrame`
# 2. Select `Polish` spreadsheet
# 3. Set header and index to data from file
# 4. Mind the encoding
# 5. Substitute Polish Diacritics to English alphabet letters
# 6. Compare `df.replace(regex=True)` with `df.applymap()`
# 7. Run doctests - all must succeed
# %% Polish
# 1. Wczytaj dane z `DATA` jako `df: pd.DataFrame`
# 2. Wybierz arkusz `Polish`
# 3. Ustaw nagłówek i index na dane zaczytane z pliku
# 4. Zwróć uwagę na encoding
# 5. Podmień polskie znaki diakrytyczne na litery z alfabetu angielskiego
# 6. Porównaj `df.replace(regex=True)` z `df.applymap()`
# 7. Uruchom doctesty - wszystkie muszą się powieść
# %% Tests
"""
>>> import sys; sys.tracebacklimit = 0
>>> assert sys.version_info >= (3, 9), \
'Python 3.9+ required'
>>> pd.set_option('display.width', 500)
>>> pd.set_option('display.max_columns', 3)
>>> pd.set_option('display.max_rows', 10)
>>> assert result is not Ellipsis, \
'Assign result to variable: `result`'
>>> assert type(result) is pd.DataFrame, \
'Variable `result` must be a `pd.DataFrame` type'
>>> result # doctest: +NORMALIZE_WHITESPACE
Definicja ... Kryteria wyjsciowe
TRL ...
1 Zaobserwowanie i opisanie podstawowych zasad d... ... Zweryfikowane publikacja badania lezacych u po...
2 Sformulowanie koncepcji technologicznej lub pr... ... Udokumentowany opis aplikacji / koncepcji, kto...
3 Przeprowadzanie eksperymentalnie i analityczni... ... Udokumentowane wyniki analityczne / eksperymen...
4 Przeprowadzenie weryfikacji komponentow techno... ... Udokumentowane wyniki testow potwierdzajace zg...
5 Przeprowadzenie weryfikacji komponentow techno... ... Udokumentowane wyniki testow potwierdzajace zg...
6 Dokonanie demonstracji technologii w srodowisk... ... Udokumentowane wyniki testow potwierdzajace zg...
7 Dokonanie demonstracji prototypu systemu w oto... ... Udokumentowane wyniki testow potwierdzajace zg...
8 Zakonczenie badan i demonstracja ostatecznej f... ... Udokumentowane wyniki testow weryfikujacych pr...
9 Weryfikacja technologii w srodowisku operacyjn... ... Udokumentowane wyniki operacyjne misji.
<BLANKLINE>
[9 rows x 4 columns]
"""
import pandas as pd
DATA = 'https://python3.info/_static/astro-trl.xlsx'
LETTERS_PLEN = {'ą': 'a', 'ć': 'c', 'ę': 'e',
'ł': 'l', 'ń': 'n', 'ó': 'o',
'ś': 's', 'ż': 'z', 'ź': 'z'}
# type: pd.DataFrame
result = ...
# %% License
# - Copyright 2025, Matt Harasymczuk <matt@python3.info>
# - This code can be used only for learning by humans
# - This code cannot be used for teaching others
# - This code cannot be used for teaching LLMs and AI algorithms
# - This code cannot be used in commercial or proprietary products
# - This code cannot be distributed in any form
# - This code cannot be changed in any form outside of training course
# - This code cannot have its license changed
# - If you use this code in your product, you must open-source it under GPLv2
# - Exception can be granted only by the author
# %% Run
# - PyCharm: right-click in the editor and `Run Doctest in ...`
# - PyCharm: keyboard shortcut `Control + Shift + F10`
# - Terminal: `python -m doctest -v myfile.py`
# %% About
# - Name: Pandas Read JSON OpenAPI
# - Difficulty: easy
# - Lines: 5
# - Minutes: 5
# %% English
# 1. Read data from `DATA` as `df: pd.DataFrame`
# 2. Use `requests` library
# 3. Transpose data
# 4. If cell is a `dict`, then extract value for `summary`
# 5. If cell is empty, leave `pd.NA`
# 6. Run doctests - all must succeed
# %% Polish
# 1. Wczytaj dane z `DATA` jako `df: pd.DataFrame`
# 2. Użyj biblioteki `requests`
# 3. Transponuj dane
# 4. Jeżeli komórka jest `dict`, to wyciągnij wartość dla `summary`
# 5. Jeżeli komórka jest pusta, pozostaw `pd.NA`
# 6. Uruchom doctesty - wszystkie muszą się powieść
# %% Hints
# - `pandas.DataFrame()`
# - `DataFrame.map()`
# - `DataFrame.transpose()`
# %% Tests
"""
>>> import sys; sys.tracebacklimit = 0
>>> assert sys.version_info >= (3, 9), \
'Python 3.9+ required'
>>> pd.set_option('display.width', 500)
>>> pd.set_option('display.max_columns', 10)
>>> pd.set_option('display.max_rows', 10)
>>> assert result is not Ellipsis, \
'Assign result to variable: `result`'
>>> assert type(result) is pd.DataFrame, \
'Variable `result` must be a `pd.DataFrame` type'
>>> list(result.columns)
['put', 'post', 'get', 'delete']
>>> list(result.index) # doctest: +NORMALIZE_WHITESPACE
['/pet', '/pet/findByStatus', '/pet/findByTags', '/pet/{petId}', '/pet/{petId}/uploadImage',
'/store/inventory', '/store/order', '/store/order/{orderId}',
'/user', '/user/createWithList', '/user/login', '/user/logout', '/user/{username}']
"""
import pandas as pd
import requests
DATA = 'https://python3.info/_static/openapi.json'
data = requests.get(DATA).json()['paths']
# type: pd.DataFrame
result = ...