4.4. Optimization Profiling
A profile is a set of statistics that describes how often and for how long various parts of the program executed
The profiler modules are designed to provide an execution profile for a given program, not for benchmarking purposes (for that, there is timeit for reasonably accurate results). This particularly applies to benchmarking Python code against C code: the profilers introduce overhead for Python code, but not for C-level functions, and so the C code would seem faster than any Python one.
4.4.1. Profilers
cProfile (CPython built-in)
PyCharm IDE
memory_profiler
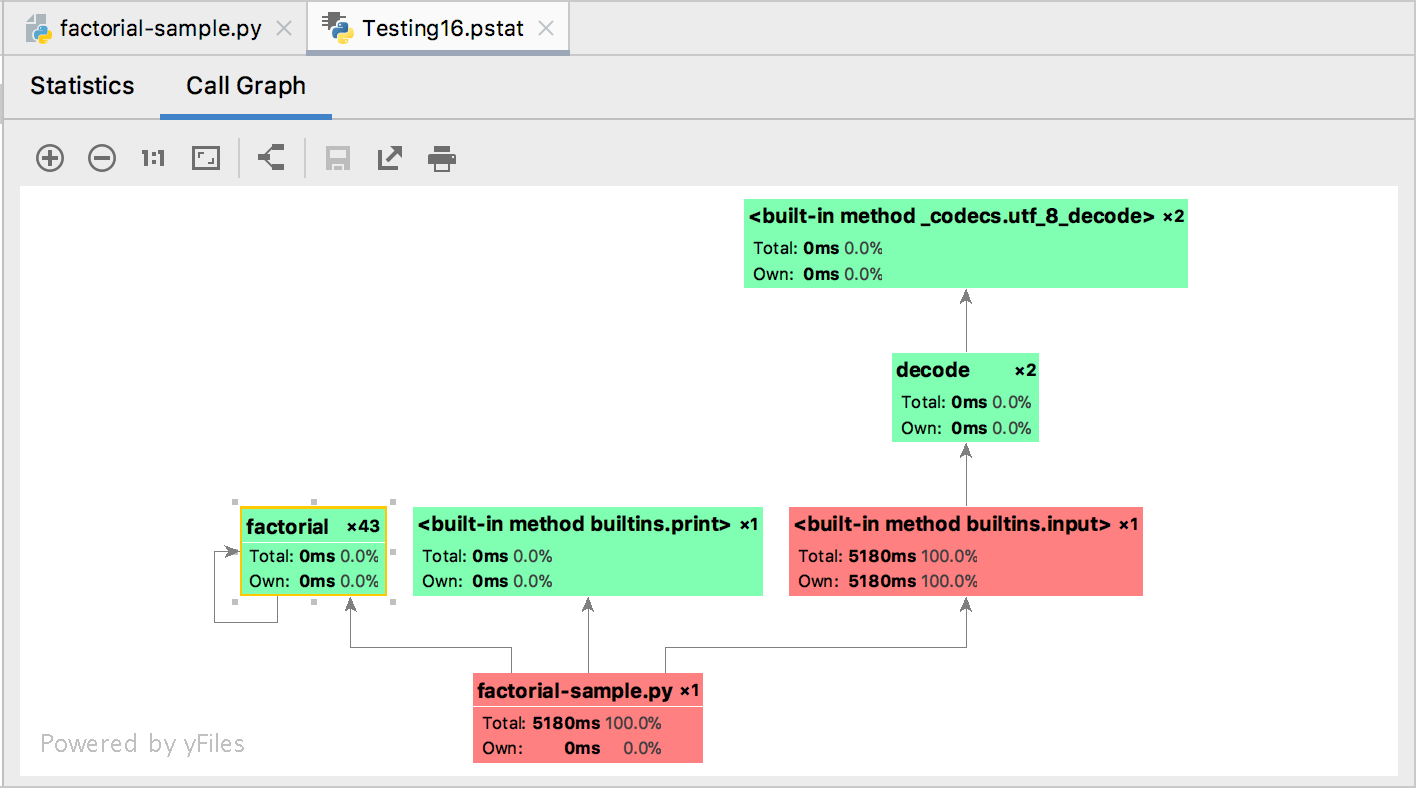
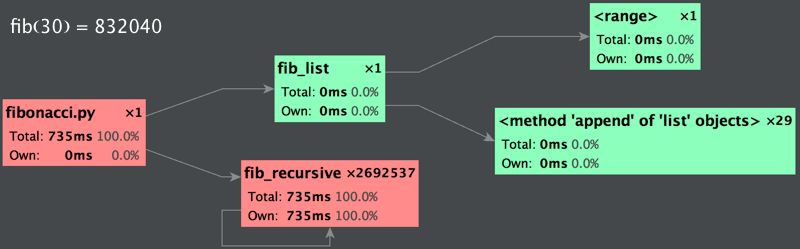
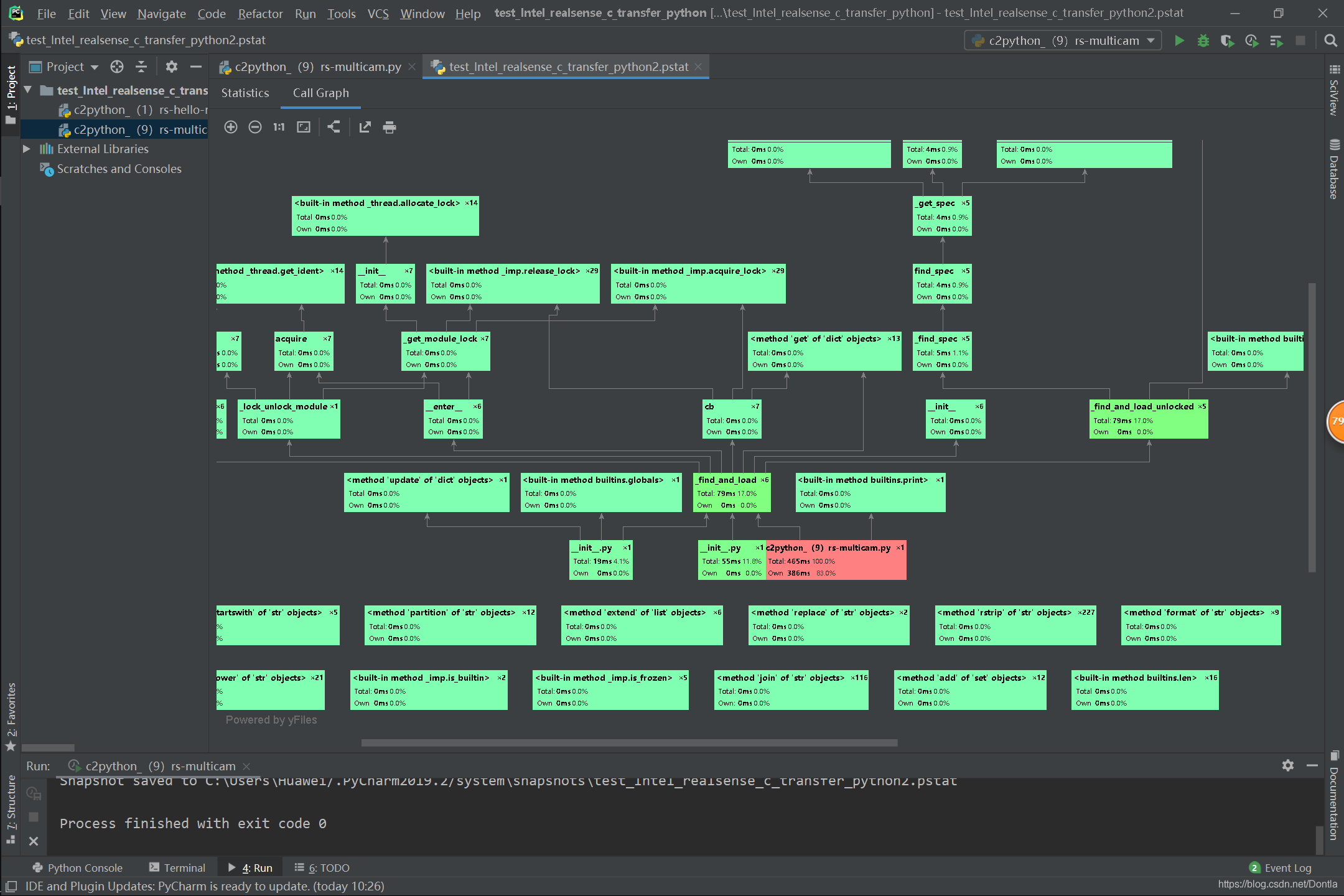
4.4.2. cProfile
>>>
... import cProfile
...
... cProfile.run('import re; re.compile("foo|bar")')
216 function calls (209 primitive calls) in 0.000 seconds
Ordered by: standard name
ncalls tottime percall cumtime percall filename:lineno(function)
1 0.000 0.000 0.000 0.000 <string>:1(<module>)
2 0.000 0.000 0.000 0.000 enum.py:284(__call__)
2 0.000 0.000 0.000 0.000 enum.py:526(__new__)
1 0.000 0.000 0.000 0.000 enum.py:836(__and__)
1 0.000 0.000 0.000 0.000 pydev_import_hook.py:16(do_import)
1 0.000 0.000 0.000 0.000 re.py:232(compile)
1 0.000 0.000 0.000 0.000 re.py:271(_compile)
1 0.000 0.000 0.000 0.000 sre_compile.py:249(_compile_charset)
1 0.000 0.000 0.000 0.000 sre_compile.py:276(_optimize_charset)
2 0.000 0.000 0.000 0.000 sre_compile.py:453(_get_iscased)
1 0.000 0.000 0.000 0.000 sre_compile.py:461(_get_literal_prefix)
1 0.000 0.000 0.000 0.000 sre_compile.py:492(_get_charset_prefix)
1 0.000 0.000 0.000 0.000 sre_compile.py:536(_compile_info)
2 0.000 0.000 0.000 0.000 sre_compile.py:595(isstring)
1 0.000 0.000 0.000 0.000 sre_compile.py:598(_code)
3/1 0.000 0.000 0.000 0.000 sre_compile.py:71(_compile)
1 0.000 0.000 0.000 0.000 sre_compile.py:759(compile)
3 0.000 0.000 0.000 0.000 sre_parse.py:111(__init__)
7 0.000 0.000 0.000 0.000 sre_parse.py:160(__len__)
18 0.000 0.000 0.000 0.000 sre_parse.py:164(__getitem__)
7 0.000 0.000 0.000 0.000 sre_parse.py:172(append)
3/1 0.000 0.000 0.000 0.000 sre_parse.py:174(getwidth)
1 0.000 0.000 0.000 0.000 sre_parse.py:224(__init__)
8 0.000 0.000 0.000 0.000 sre_parse.py:233(__next)
2 0.000 0.000 0.000 0.000 sre_parse.py:249(match)
6 0.000 0.000 0.000 0.000 sre_parse.py:254(get)
1 0.000 0.000 0.000 0.000 sre_parse.py:286(tell)
1 0.000 0.000 0.000 0.000 sre_parse.py:417(_parse_sub)
2 0.000 0.000 0.000 0.000 sre_parse.py:475(_parse)
1 0.000 0.000 0.000 0.000 sre_parse.py:76(__init__)
2 0.000 0.000 0.000 0.000 sre_parse.py:81(groups)
1 0.000 0.000 0.000 0.000 sre_parse.py:903(fix_flags)
1 0.000 0.000 0.000 0.000 sre_parse.py:919(parse)
1 0.000 0.000 0.000 0.000 {built-in method _sre.compile}
1 0.000 0.000 0.000 0.000 {built-in method builtins.__import__}
1 0.000 0.000 0.000 0.000 {built-in method builtins.exec}
25 0.000 0.000 0.000 0.000 {built-in method builtins.isinstance}
29/26 0.000 0.000 0.000 0.000 {built-in method builtins.len}
2 0.000 0.000 0.000 0.000 {built-in method builtins.max}
9 0.000 0.000 0.000 0.000 {built-in method builtins.min}
6 0.000 0.000 0.000 0.000 {built-in method builtins.ord}
48 0.000 0.000 0.000 0.000 {method 'append' of 'list' objects}
1 0.000 0.000 0.000 0.000 {method 'disable' of '_lsprof.Profiler' objects}
5 0.000 0.000 0.000 0.000 {method 'find' of 'bytearray' objects}
1 0.000 0.000 0.000 0.000 {method 'items' of 'dict' objects}
Name |
Description |
---|---|
ncalls |
for the number of calls |
tottime |
for the total time spent in the given function (and excluding time made in calls to sub-functions) |
percall |
is the quotient of tottime divided by ncalls |
cumtime |
is the cumulative time spent in this and all subfunctions (from invocation till exit) |
percall |
is the quotient of cumtime divided by primitive calls |
filename:lineno(function) |
provides the respective data of each function |
Name |
Description |
---|---|
calls |
call count |
cumulative |
cumulative time |
cumtime |
cumulative time |
file |
file name |
filename |
file name |
module |
file name |
ncalls |
call count |
pcalls |
primitive call count |
line |
line number |
name |
function name |
nfl |
name/file/line |
stdname |
standard name |
time |
internal time |
tottime |
internal time |
$ python -m cProfile [-o output_file] [-s sort_order] FILE.py
4.4.3. Memory Profiler
Memory Profiler is a Python module for monitoring memory consumption of a process as well as line-by-line analysis of memory consumption for Python programs.
pip install memory-profiler
python -m memory_profiler myfile.py
from memory_profiler import profile, memory_usage
@profile
usage = memory_usage((func, (arg1, arg2), {'kwarg1': 'val'}))
python -m mprof run myfile.py
python -m mprof plot
$ pip install memory-profiler
$ python -m memory_profiler myfile.py
$ python -m mprof run myfile.py
$ python -m mprof plot
4.4.4. Yappi
Yappi is a high-performance statistical profiler for Python. It is well-suited for profiling multi-threaded applications and handling partial loads of a program.
pip install yappi
python -m yappi myfile.py
yappi.set_clock_type("cpu")
# Useset_clock_type("wall")
for wall timeyappi.start()
yappi.get_func_stats().print_all()
yappi.get_thread_stats().print_all()
$ pip install yappi
$ python -m yappi myfile.py
>>>
... import yappi
...
... def a():
... for _ in range(1_000): # do something CPU heavy
... pass
...
... yappi.set_clock_type("cpu") # Use set_clock_type("wall") for wall time
... yappi.start()
... a()
...
... yappi.get_func_stats().print_all()
... yappi.get_thread_stats().print_all()
Clock type: CPU
Ordered by: totaltime, desc
name ncall tsub ttot tavg doc.py:5 a 1 0.117907 0.117907 0.117907
name id tid ttot scnt _MainThread 0 139867147315008 0.118297 1
>>>
... import yappi
... import time
... import threading
...
... _NTHREAD = 3
...
...
... def _work(n):
... time.sleep(n * 0.1)
...
...
... yappi.start()
...
... threads = []
... # generate _NTHREAD threads
... for i in range(_NTHREAD):
... t = threading.Thread(target=_work, args=(i + 1, ))
... t.start()
... threads.append(t)
... # wait all threads to finish
... for t in threads:
... t.join()
...
... yappi.stop()
...
... # retrieve thread stats by their thread id (given by yappi)
... threads = yappi.get_thread_stats()
... for thread in threads:
... print("Function stats for (%s) (%d)" % (thread.name, thread.id)
... ) # it is the Thread.__class__.__name__
... yappi.get_func_stats(ctx_id=thread.id).print_all()
Function stats for (Thread) (3)
name ncall tsub ttot tavg ..hon3.7/threading.py:859 Thread.run 1 0.000017 0.000062 0.000062 doc3.py:8 _work 1 0.000012 0.000045 0.000045
Function stats for (Thread) (2)
name ncall tsub ttot tavg ..hon3.7/threading.py:859 Thread.run 1 0.000017 0.000065 0.000065 doc3.py:8 _work 1 0.000010 0.000048 0.000048
Function stats for (Thread) (1)
name ncall tsub ttot tavg ..hon3.7/threading.py:859 Thread.run 1 0.000010 0.000043 0.000043 doc3.py:8 _work 1 0.000006 0.000033 0.000033
Async application:
>>>
... import asyncio
... import yappi
...
... async def foo():
... await asyncio.sleep(1.0)
... await baz()
... await asyncio.sleep(0.5)
...
... async def bar():
... await asyncio.sleep(2.0)
...
... async def baz():
... await asyncio.sleep(1.0)
...
...
... yappi.set_clock_type("WALL")
...
... with yappi.run():
... asyncio.run(foo())
... asyncio.run(bar())
...
... yappi.get_func_stats().print_all()
Clock type: WALL
Ordered by: totaltime, desc
name ncall tsub ttot tavg doc4.py:5 foo 1 0.000030 2.503808 2.503808 doc4.py:11 bar 1 0.000012 2.002492 2.002492 doc4.py:15 baz 1 0.000013 1.001397 1.001397