4.1. Optimization Complexity
Computational Complexity
Memory Complexity
Cognitive Complexity
Cyclomatic Complexity
Big O notation [1]
https://dev.to/alvbarros/learn-big-o-notation-once-and-for-all-hm9
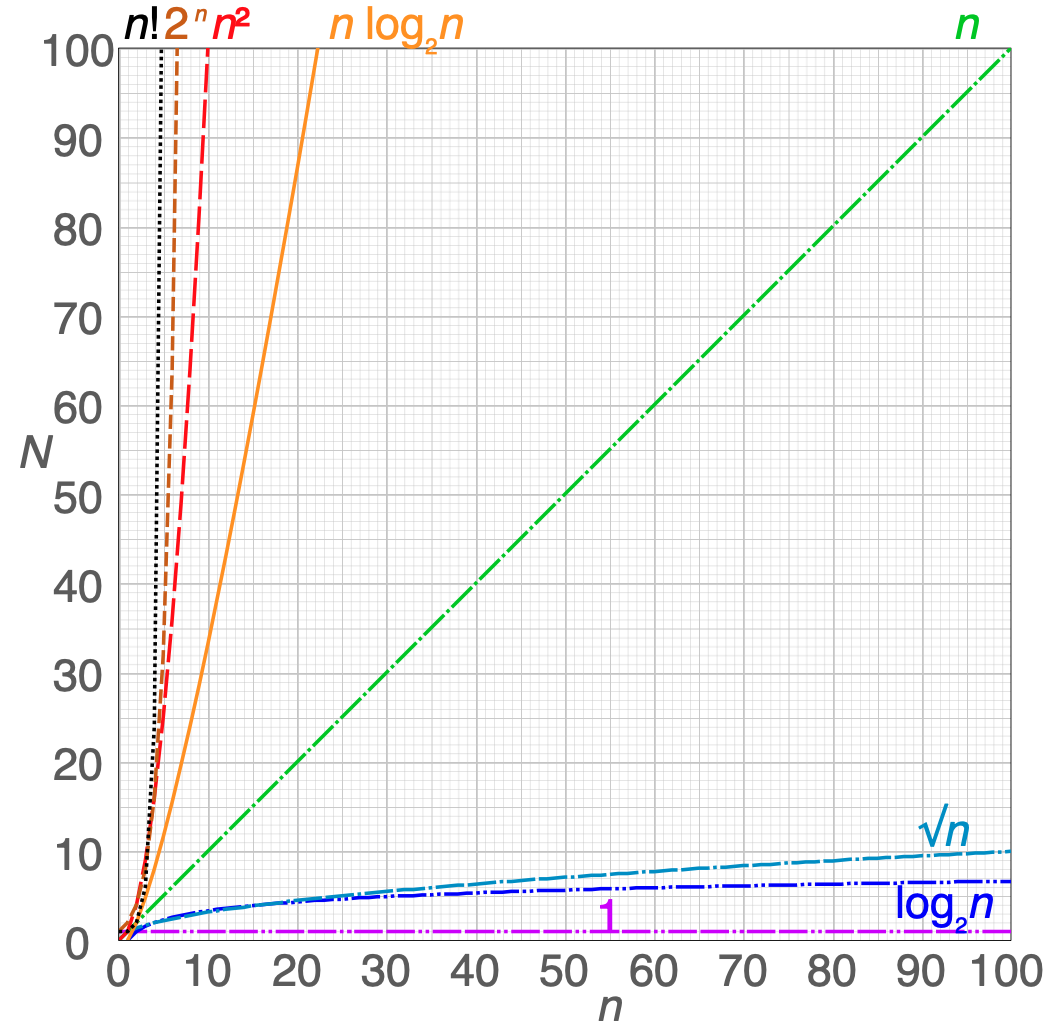
4.1.1. Computational Complexity
4.1.2. Memory Complexity
4.1.3. Cognitive Complexity
Measure of how difficult the application is to understand
Measure of how hard the control flow of a function is to understand
Functions with high Cognitive Complexity will be difficult to maintain.
4.1.4. Cyclomatic Complexity
Measures the minimum number of test cases required for full test coverage.
4.1.5. Big O notation
Most common:
O(sqrt_n)
O(log_n)
O(log2_n)
O(1)
O(n)
O(nlog2_n)
O(n^2)
O(2^n)
O(n!)
Running time (T(n)) |
Name |
Example algorithms |
---|---|---|
|
constant time |
Finding the median value in a sorted array of numbersCalculating (−1)n |
|
inverse Ackermann time |
Amortized time per operation using a disjoint set |
|
iterated logarithmic time |
Distributed coloring of cycles |
|
log-logarithmic |
Amortized time per operation using a bounded priority queue |
|
logarithmic time |
Binary search |
|
polylogarithmic time |
|
|
fractional power |
Searching in a kd-tree |
|
linear time |
Finding the smallest or largest item in an unsorted array, Kadane's algorithm, linear search |
|
n log-star n time |
Seidel's polygon triangulation algorithm |
|
linearithmic time |
Fastest possible comparison sort; Fast Fourier transform |
|
quasilinear time |
|
|
quadratic time |
Bubble sort; Insertion sort; Direct convolution |
|
cubic time |
Naive multiplication of two n×n matrices. Calculating partial correlation |
|
polynomial time |
Karmarkar's algorithm for linear programming; AKS primality test |
|
quasi-polynomial time |
Best-known O(log2 n)-approximation algorithm for the directed Steiner tree problem |
|
sub-exponential time |
Best-known algorithm for integer factorization; formerly-best algorithm for graph isomorphism |
|
sub-exponential time |
Best-known algorithm for integer factorization; formerly-best algorithm for graph isomorphism |
|
exponential time (with linear exponent) |
Solving the traveling salesman problem using dynamic programming |
|
exponential time |
Solving matrix chain multiplication via brute-force search |
|
factorial time |
Solving the traveling salesman problem via brute-force search |
|
double exponential time |
Deciding the truth of a given statement in Presburger arithmetic |
4.1.6. References
4.1.7. Assignments
# %% License
# - Copyright 2025, Matt Harasymczuk <matt@python3.info>
# - This code can be used only for learning by humans
# - This code cannot be used for teaching others
# - This code cannot be used for teaching LLMs and AI algorithms
# - This code cannot be used in commercial or proprietary products
# - This code cannot be distributed in any form
# - This code cannot be changed in any form outside of training course
# - This code cannot have its license changed
# - If you use this code in your product, you must open-source it under GPLv2
# - Exception can be granted only by the author
# %% Run
# - PyCharm: right-click in the editor and `Run Doctest in ...`
# - PyCharm: keyboard shortcut `Control + Shift + F10`
# - Terminal: `python -m doctest -v myfile.py`
# %% About
# - Name: Performance Compexity Segmentation
# - Difficulty: easy
# - Lines: 8
# - Minutes: 5
# %% English
# 1. Count occurrences of each group
# 2. Define groups:
# - `small` - numbers in range [0-3)
# - `medium` - numbers in range [3-7)
# - `large` - numbers in range [7-10)
# 3. Print `result: dict[str, int]`:
# - key - group
# - value - number of occurrences
# 4. Run doctests - all must succeed
# %% Polish
# 1. Policz wystąpienia każdej z group
# 2. Zdefiniuj grupy
# - `small` - liczby z przedziału <0-3)
# - `medium` - liczby z przedziału <3-7)
# - `large` - liczby z przedziału <7-10)
# 3. Wypisz `result: dict[str, int]`:
# - klucz - grupa
# - wartość - liczba wystąpień
# 4. Uruchom doctesty - wszystkie muszą się powieść
# %% Tests
"""
>>> import sys; sys.tracebacklimit = 0
>>> assert sys.version_info >= (3, 9), \
'Python 3.9+ required'
>>> type(result)
<class 'dict'>
>>> assert all(type(x) is str for x in result.keys())
>>> assert all(type(x) is int for x in result.values())
>>> result
{'small': 16, 'medium': 19, 'large': 15}
"""
DATA = [
1, 4, 6, 7, 4, 4, 4, 5, 1, 7, 0,
0, 6, 5, 0, 0, 9, 7, 0, 4, 4, 8,
2, 4, 0, 0, 1, 9, 1, 7, 8, 8, 9,
1, 3, 5, 6, 8, 2, 8, 1, 3, 9, 5,
4, 8, 1, 9, 6, 3,
]
# dict[str,int] number of digit occurrences in segments
result = {'small': 0, 'medium': 0, 'large': 0}
# %% License
# - Copyright 2025, Matt Harasymczuk <matt@python3.info>
# - This code can be used only for learning by humans
# - This code cannot be used for teaching others
# - This code cannot be used for teaching LLMs and AI algorithms
# - This code cannot be used in commercial or proprietary products
# - This code cannot be distributed in any form
# - This code cannot be changed in any form outside of training course
# - This code cannot have its license changed
# - If you use this code in your product, you must open-source it under GPLv2
# - Exception can be granted only by the author
# %% Run
# - PyCharm: right-click in the editor and `Run Doctest in ...`
# - PyCharm: keyboard shortcut `Control + Shift + F10`
# - Terminal: `python -m doctest -v myfile.py`
# %% About
# - Name: Performance Compexity UniqueKeys
# - Difficulty: easy
# - Lines: 3
# - Minutes: 5
# %% English
# 1. Collect unique keys from all rows in one sequence `result`
# 2. Run doctests - all must succeed
# %% Polish
# 1. Zbierz unikalne klucze z wszystkich wierszy w jednej sekwencji `result`
# 2. Uruchom doctesty - wszystkie muszą się powieść
# %% Hints
# - `row.keys()`
# - Compare solutions with `Micro-benchmarking`
# %% Tests
"""
>>> import sys; sys.tracebacklimit = 0
>>> assert sys.version_info >= (3, 9), \
'Python 3.9+ required'
>>> result is not Ellipsis
True
>>> type(result) in (set, list, tuple, frozenset)
True
>>> sorted(result)
['petal_length', 'petal_width', 'sepal_length', 'sepal_width', 'species']
"""
DATA = [
{'sepal_length': 5.1, 'sepal_width': 3.5, 'species': 'setosa'},
{'petal_length': 4.1, 'petal_width': 1.3, 'species': 'versicolor'},
{'sepal_length': 6.3, 'petal_width': 1.8, 'species': 'virginica'},
{'sepal_length': 5.0, 'petal_width': 0.2, 'species': 'setosa'},
{'sepal_width': 2.8, 'petal_length': 4.1, 'species': 'versicolor'},
{'sepal_width': 2.9, 'petal_width': 1.8, 'species': 'virginica'},
]
# Unique keys from DATA dicts
# type: set[str]
result = ...
# %% License
# - Copyright 2025, Matt Harasymczuk <matt@python3.info>
# - This code can be used only for learning by humans
# - This code cannot be used for teaching others
# - This code cannot be used for teaching LLMs and AI algorithms
# - This code cannot be used in commercial or proprietary products
# - This code cannot be distributed in any form
# - This code cannot be changed in any form outside of training course
# - This code cannot have its license changed
# - If you use this code in your product, you must open-source it under GPLv2
# - Exception can be granted only by the author
# %% Run
# - PyCharm: right-click in the editor and `Run Doctest in ...`
# - PyCharm: keyboard shortcut `Control + Shift + F10`
# - Terminal: `python -m doctest -v myfile.py`
# %% About
# - Name: Performance Compexity SplitTrainTest
# - Difficulty: easy
# - Lines: 4
# - Minutes: 5
# %% English
# 1. Using List Comprehension split `DATA` into:
# - `features_train: list[tuple]` - 60% of first features in `DATA`
# - `features_test: list[tuple]` - 40% of last features in `DATA`
# - `labels_train: list[str]` - 60% of first labels in `DATA`
# - `labels_test: list[str]` - 40% of last labels in `DATA`
# 2. In order to do so, calculate pivot point:
# - length of `DATA` times given percent (60% = 0.6)
# - remember, that slice indicies must be `int`, not `float`
# - for example: if dataset has 10 rows, then 6 rows will be for
# training, and 4 rows for test
# 3. Run doctests - all must succeed
# %% Polish
# 1. Używając List Comprehension podziel `DATA` na:
# - `features_train: list[tuple]` - 60% pierwszych features w `DATA`
# - `features_test: list[tuple]` - 40% ostatnich features w `DATA`
# - `labels_train: list[str]` - 60% pierwszych labels w `DATA`
# - `labels_test: list[str]` - 40% ostatnich labels w `DATA`
# 2. Aby to zrobić, wylicz punkt podziału:
# - długość `DATA` razy zadany procent (60% = 0.6)
# - pamiętaj, że indeksy slice muszą być `int` a nie `float`
# - na przykład: if zbiór danych ma 10 wierszy, to 6 wierszy będzie
# do treningu, a 4 do testów
# 3. Uruchom doctesty - wszystkie muszą się powieść
# %% Tests
"""
>>> import sys; sys.tracebacklimit = 0
>>> assert sys.version_info >= (3, 9), \
'Python 3.9+ required'
>>> assert type(features_train) is list, \
'make sure features_train is a list'
>>> assert type(features_test) is list, \
'make sure features_test is a list'
>>> assert type(labels_train) is list, \
'make sure labels_train is a list'
>>> assert type(labels_test) is list, \
'make sure labels_test is a list'
>>> assert all(type(x) is tuple for x in features_train), \
'all elements in features_train should be tuple'
>>> assert all(type(x) is tuple for x in features_test), \
'all elements in features_test should be tuple'
>>> assert all(type(x) is str for x in labels_train), \
'all elements in labels_train should be str'
>>> assert all(type(x) is str for x in labels_test), \
'all elements in labels_test should be str'
>>> features_train # doctest: +NORMALIZE_WHITESPACE
[(5.8, 2.7, 5.1, 1.9),
(5.1, 3.5, 1.4, 0.2),
(5.7, 2.8, 4.1, 1.3),
(6.3, 2.9, 5.6, 1.8),
(6.4, 3.2, 4.5, 1.5),
(4.7, 3.2, 1.3, 0.2)]
>>> features_test # doctest: +NORMALIZE_WHITESPACE
[(7.0, 3.2, 4.7, 1.4),
(7.6, 3.0, 6.6, 2.1),
(4.9, 3.0, 1.4, 0.2),
(4.9, 2.5, 4.5, 1.7)]
>>> labels_train
['virginica', 'setosa', 'versicolor', 'virginica', 'versicolor', 'setosa']
>>> labels_test
['versicolor', 'virginica', 'setosa', 'virginica']
"""
DATA = [
('sepal_length', 'sepal_width', 'petal_length', 'petal_width', 'species'),
(5.8, 2.7, 5.1, 1.9, 'virginica'),
(5.1, 3.5, 1.4, 0.2, 'setosa'),
(5.7, 2.8, 4.1, 1.3, 'versicolor'),
(6.3, 2.9, 5.6, 1.8, 'virginica'),
(6.4, 3.2, 4.5, 1.5, 'versicolor'),
(4.7, 3.2, 1.3, 0.2, 'setosa'),
(7.0, 3.2, 4.7, 1.4, 'versicolor'),
(7.6, 3.0, 6.6, 2.1, 'virginica'),
(4.9, 3.0, 1.4, 0.2, 'setosa'),
(4.9, 2.5, 4.5, 1.7, 'virginica'),
]
ratio = 0.6
header, *rows = DATA
split = int(len(rows) * ratio)
features_train = ...
features_test = ...
labels_train = ...
labels_test = ...
# %% License
# - Copyright 2025, Matt Harasymczuk <matt@python3.info>
# - This code can be used only for learning by humans
# - This code cannot be used for teaching others
# - This code cannot be used for teaching LLMs and AI algorithms
# - This code cannot be used in commercial or proprietary products
# - This code cannot be distributed in any form
# - This code cannot be changed in any form outside of training course
# - This code cannot have its license changed
# - If you use this code in your product, you must open-source it under GPLv2
# - Exception can be granted only by the author
# %% Run
# - PyCharm: right-click in the editor and `Run Doctest in ...`
# - PyCharm: keyboard shortcut `Control + Shift + F10`
# - Terminal: `python -m doctest -v myfile.py`
# %% About
# - Name: Unpack Parameters Define
# - Difficulty: easy
# - Lines: 4
# - Minutes: 5
# %% English
# 1. Create function `mean()`, which calculates arithmetic mean
# 2. Function can have arbitrary number of positional arguments
# 3. Non-functional requirements:
# - Do not import any libraries and modules
# - Use builtin functions `sum()` and `len()`
# 4. Run doctests - all must succeed
# %% Polish
# 1. Napisz funkcję `mean()`, wyliczającą średnią arytmetyczną
# 2. Funkcja przyjmuje dowolną ilość pozycyjnych argumentów
# 3. Wymagania niefunkcjonalne:
# - Nie importuj żadnych bibliotek i modułów
# - Użyj wbudowanych funckji `sum()` i `len()`
# 4. Uruchom doctesty - wszystkie muszą się powieść
# %% Hints
# - `raise ValueError('error message')`
# - `sum(...) / len(...)`
# %% Tests
"""
>>> import sys; sys.tracebacklimit = 0
>>> assert sys.version_info >= (3, 9), \
'Python 3.9+ required'
>>> mean(1)
1.0
>>> mean(1, 2)
1.5
>>> mean(1, 2, 3)
2.0
>>> mean(1, 2, 3, 4)
2.5
>>> mean()
Traceback (most recent call last):
ValueError: At least one argument is required
"""