10.1. K-Means Clustering
A loose definition of clustering could be "the process of organizing objects into groups whose members are similar in some way". A cluster is therefore a collection of objects which are "similar" between them and are "dissimilar" to the objects belonging to other clusters.
Clustering algorithms are usually unsupervised.
How many groups (clusters) you want your data to be in?
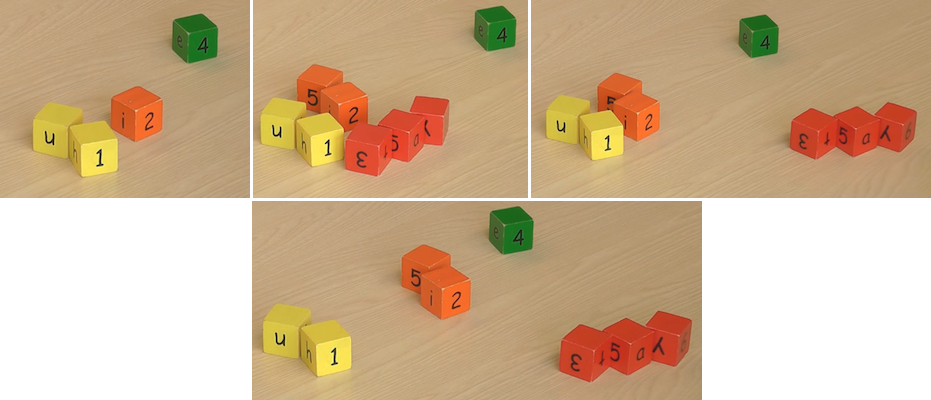
Figure 10.3. Clustering (grouping) data.
When you sort data in unsupervised learning you quite often ends up with looking good at the beginning.
When you look at data from different perspective it's not so great.
Algorithms only work when you tell them, how many groups you want data in.
10.1.1. Przykłady zastosowania
pixels colors
patients in hospital: how sick they are
cars: new, used
jobs: different kinds
10.1.2. Algorytmy klastrowania
Method name |
Parameters |
Scalability |
Usecase |
Geometry (metric used) |
---|---|---|---|---|
K-Means |
number of clusters |
Very large |
General-purpose, even cluster size, flat geometry, not too many clusters |
Distances between points |
Affinity propagation |
damping, sample preference |
Not scalable with n_samples |
Many clusters, uneven cluster size, non-flat geometry |
Graph distance (e.g. nearest-neighbor graph) |
Mean-shift |
bandwidth |
Not scalable with |
Many clusters, uneven cluster size, non-flat geometry |
Distances between points |
Spectral clustering |
number of clusters |
Medium |
Few clusters, even cluster size, non-flat geometry |
Graph distance (e.g. nearest-neighbor graph) |
Ward hierarchical clustering |
number of clusters |
Large |
Many clusters, possibly connectivity constraints |
Distances between points |
Agglomerative clustering |
number of clusters, linkage type, distance |
Large |
Many clusters, possibly connectivity constraints, non Euclidean distances |
Any pairwise distance |
DBSCAN |
neighborhood size |
Very large |
Non-flat geometry, uneven cluster sizes |
Distances between nearest points |
Gaussian mixtures |
many |
Not scalable |
Flat geometry, good for density estimation |
Mahalanobis distances to centers |
Birch |
branching factor, threshold, optional global clusterer. |
Large |
Large dataset, outlier removal, data reduction. |
Euclidean distance between points |
10.1.3. Similarities
statistical
algebraical
geometrical
10.1.4. Flat Clustering
Clustering algorithms group a set of documents into subsets or clusters . The algorithms' goal is to create clusters that are coherent internally, but clearly different from each other. In other words, documents within a cluster should be as similar as possible; and documents in one cluster should be as dissimilar as possible from documents in other clusters.
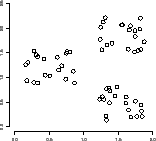
10.1.5. K-means
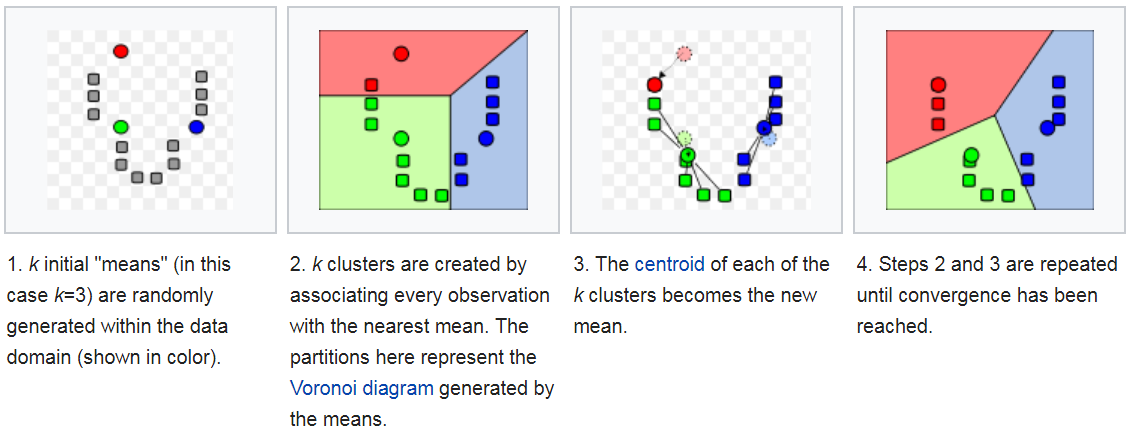
K-means Convergence:
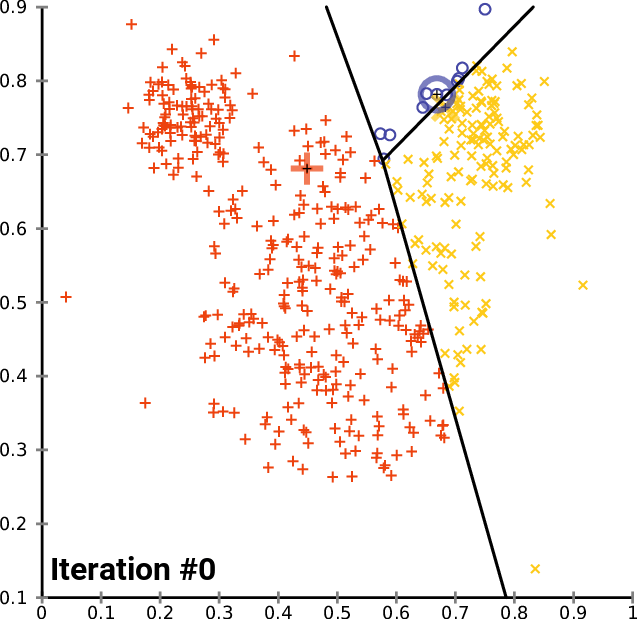
10.1.6. Hierarchical Clustering
Hierarchical clustering is where you build a cluster tree (a dendrogram) to represent data, where each group (or "node") is linked to two or more successor groups. The groups are nested and organized as a tree, which ideally ends up as a meaningful classification scheme.
Each node in the cluster tree contains a group of similar data; Nodes are placed on the graph next to other, similar nodes. Clusters at one level are joined with clusters in the next level up, using a degree of similarity; The process carries on until all nodes are in the tree, which gives a visual snapshot of the data contained in the whole set. The total number of clusters is not predetermined before you start the tree creation.
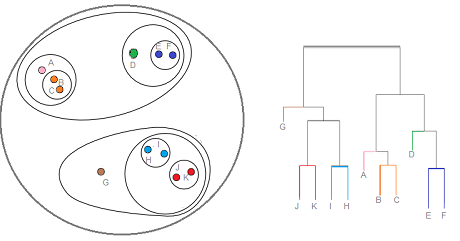
10.1.7. Porównanie algorytmów
Porównanie algorytmów klastrowania
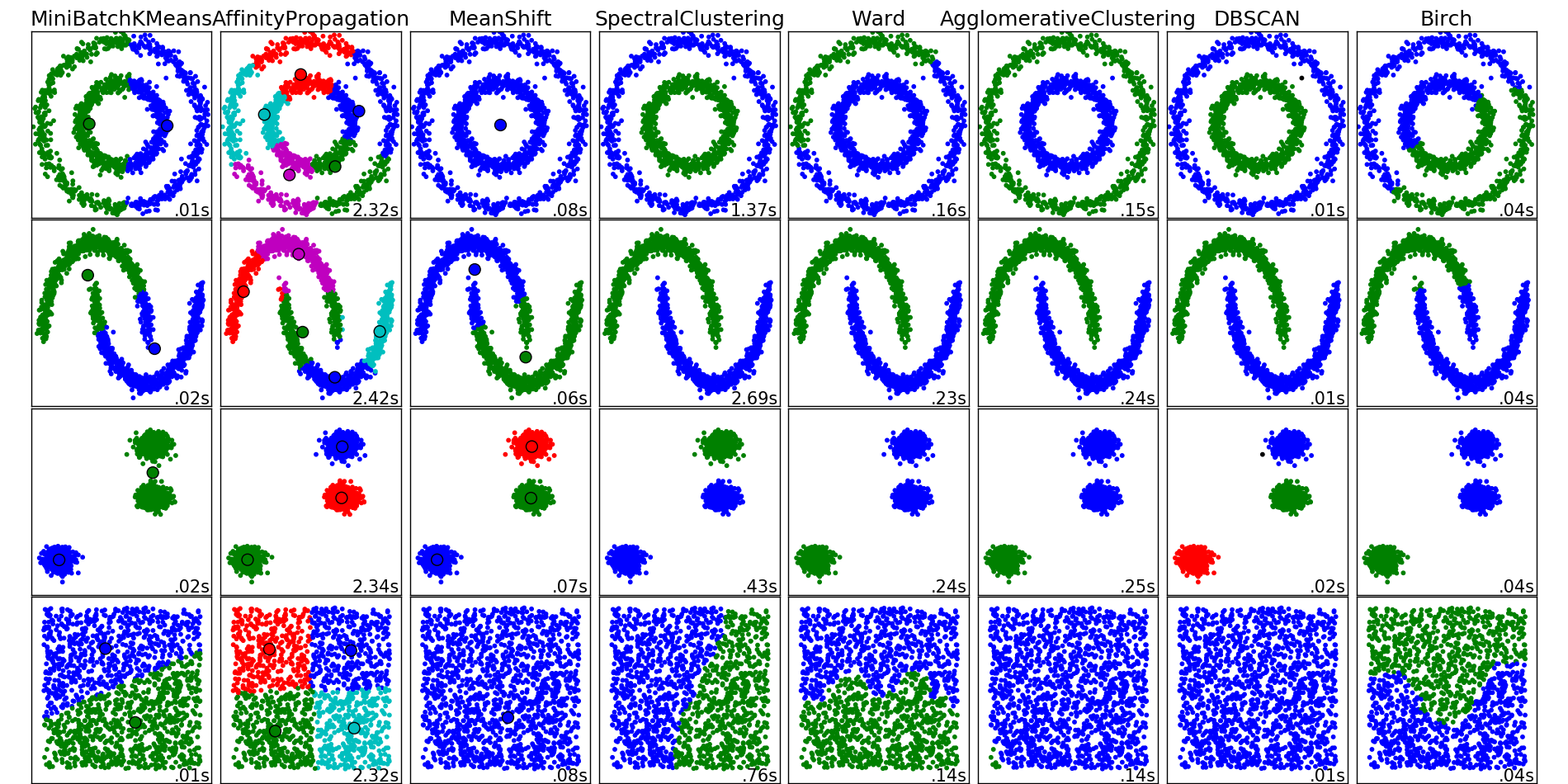
import time
import numpy as np
import matplotlib.pyplot as plt
from sklearn import cluster, datasets
from sklearn.neighbors import kneighbors_graph
from sklearn.preprocessing import StandardScaler
np.random.seed(0)
# Generate datasets. We choose the size big enough to see the scalability
# of the algorithms, but not too big to avoid too long running times
n_samples = 1500
noisy_circles = datasets.make_circles(n_samples=n_samples, factor=.5,
noise=.05)
noisy_moons = datasets.make_moons(n_samples=n_samples, noise=.05)
blobs = datasets.make_blobs(n_samples=n_samples, random_state=8)
no_structure = np.random.rand(n_samples, 2), None
colors = np.array([x for x in 'bgrcmykbgrcmykbgrcmykbgrcmyk'])
colors = np.hstack([colors] * 20)
clustering_names = [
'MiniBatchKMeans', 'AffinityPropagation', 'MeanShift',
'SpectralClustering', 'Ward', 'AgglomerativeClustering',
'DBSCAN', 'Birch']
plt.figure(figsize=(len(clustering_names) * 2 + 3, 9.5))
plt.subplots_adjust(left=.02, right=.98, bottom=.001, top=.96, wspace=.05,
hspace=.01)
plot_num = 1
datasets = [noisy_circles, noisy_moons, blobs, no_structure]
for i_dataset, dataset in enumerate(datasets):
X, y = dataset
# normalize dataset for easier parameter selection
X = StandardScaler().fit_transform(X)
# estimate bandwidth for mean shift
bandwidth = cluster.estimate_bandwidth(X, quantile=0.3)
# connectivity matrix for structured Ward
connectivity = kneighbors_graph(X, n_neighbors=10, include_self=False)
# make connectivity symmetric
connectivity = 0.5 * (connectivity + connectivity.T)
# create clustering estimators
ms = cluster.MeanShift(bandwidth=bandwidth, bin_seeding=True)
two_means = cluster.MiniBatchKMeans(n_clusters=2)
ward = cluster.AgglomerativeClustering(n_clusters=2, linkage='ward',
connectivity=connectivity)
spectral = cluster.SpectralClustering(n_clusters=2,
eigen_solver='arpack',
affinity="nearest_neighbors")
dbscan = cluster.DBSCAN(eps=.2)
affinity_propagation = cluster.AffinityPropagation(damping=.9,
preference=-200)
average_linkage = cluster.AgglomerativeClustering(
linkage="average", affinity="cityblock", n_clusters=2,
connectivity=connectivity)
birch = cluster.Birch(n_clusters=2)
clustering_algorithms = [
two_means, affinity_propagation, ms, spectral, ward, average_linkage,
dbscan, birch]
for name, algorithm in zip(clustering_names, clustering_algorithms):
# predict cluster memberships
t0 = time.time()
algorithm.fit(X)
t1 = time.time()
if hasattr(algorithm, 'labels_'):
y_pred = algorithm.labels_.astype(np.int)
else:
y_pred = algorithm.predict(X)
# plot
plt.subplot(4, len(clustering_algorithms), plot_num)
if i_dataset == 0:
plt.title(name, size=18)
plt.scatter(X[:, 0], X[:, 1], color=colors[y_pred].tolist(), s=10)
if hasattr(algorithm, 'cluster_centers_'):
centers = algorithm.cluster_centers_
center_colors = colors[:len(centers)]
plt.scatter(centers[:, 0], centers[:, 1], s=100, c=center_colors)
plt.xlim(-2, 2)
plt.ylim(-2, 2)
plt.xticks(())
plt.yticks(())
plt.text(.99, .01, ('%.2fs' % (t1 - t0)).lstrip('0'),
transform=plt.gca().transAxes, size=15,
horizontalalignment='right')
plot_num += 1
plt.show() # doctest: +SKIP
10.1.8. Przykład praktyczny
10.1.9. K-means Clustering dla zbioru Iris
import numpy as np
import matplotlib.pyplot as plt
# Though the following import is not directly being used, it is required
# for 3D projection to work
from mpl_toolkits.mplot3d import Axes3D
from sklearn.cluster import KMeans
from sklearn import datasets
np.random.seed(5)
centers = [[1, 1], [-1, -1], [1, -1]]
iris = datasets.load_iris()
X = iris.data
y = iris.target
estimators = [
('k_means_iris_8', KMeans(n_clusters=8)),
('k_means_iris_3', KMeans(n_clusters=3)),
('k_means_iris_bad_init', KMeans(n_clusters=3, n_init=1, init='random')),
]
fignum = 1
titles = ['8 clusters', '3 clusters', '3 clusters, bad initialization']
for name, est in estimators:
fig = plt.figure(fignum, figsize=(4, 3))
ax = Axes3D(fig, rect=[0, 0, .95, 1], elev=48, azim=134)
est.fit(X)
labels = est.labels_
ax.scatter(X[:, 3], X[:, 0], X[:, 2], c=labels.astype(np.float), edgecolor='k')
ax.w_xaxis.set_ticklabels([])
ax.w_yaxis.set_ticklabels([])
ax.w_zaxis.set_ticklabels([])
ax.set_xlabel('petal_width')
ax.set_ylabel('sepal_length')
ax.set_zlabel('petal_length')
ax.set_title(titles[fignum - 1])
ax.dist = 12
fignum = fignum + 1
# Plot the ground truth
fig = plt.figure(fignum, figsize=(4, 3))
ax = Axes3D(fig, rect=[0, 0, .95, 1], elev=48, azim=134)
for name, label in [('Setosa', 0),
('Versicolor', 1),
('Virginica', 2)]:
ax.text3D(X[y == label, 3].mean(),
X[y == label, 0].mean(),
X[y == label, 2].mean() + 2, name,
horizontalalignment='center',
bbox=dict(alpha=.2, edgecolor='w', facecolor='w'))
# Reorder the labels to have colors matching the cluster results
y = np.choose(y, [1, 2, 0]).astype(np.float)
ax.scatter(X[:, 3], X[:, 0], X[:, 2], c=y, edgecolor='k')
ax.w_xaxis.set_ticklabels([])
ax.w_yaxis.set_ticklabels([])
ax.w_zaxis.set_ticklabels([])
ax.set_xlabel('petal_width')
ax.set_ylabel('sepal_length')
ax.set_zlabel('petal_length')
ax.set_title('Ground Truth')
ax.dist = 12
plt.show() # doctest: +SKIP
10.1.10. Assignments
10.1.10.1. Klastrowanie zbioru Iris
- About:
Name: Klastrowanie zbioru Iris
Difficulty: medium
Lines: 30
Minutes: 13
- License:
Copyright 2025, Matt Harasymczuk <matt@python3.info>
This code can be used only for learning by humans (self-education)
This code cannot be used for teaching others (trainings, bootcamps, etc.)
This code cannot be used for teaching LLMs and AI algorithms
This code cannot be used in commercial or proprietary products
This code cannot be distributed in any form
This code cannot be changed in any form outside of training course
This code cannot have its license changed
If you use this code in your product, you must open-source it under GPLv2
Exception can be granted only by the author (Matt Harasymczuk)
- English:
- TODO: English Translation
Run doctests - all must succeed
- Polish:
Dla zbioru Iris dokonaj klastrowania za pomocą algorytmu
KMeans
z bibliotekisklearn
.Dla jakiego hiperparametru
n_clusters
osiągniemy największe accuracy?Zwizualizuj graficznie rozwiązanie problemu
Uruchom doctesty - wszystkie muszą się powieść